Automated AI Equity Research with LlamaIndex
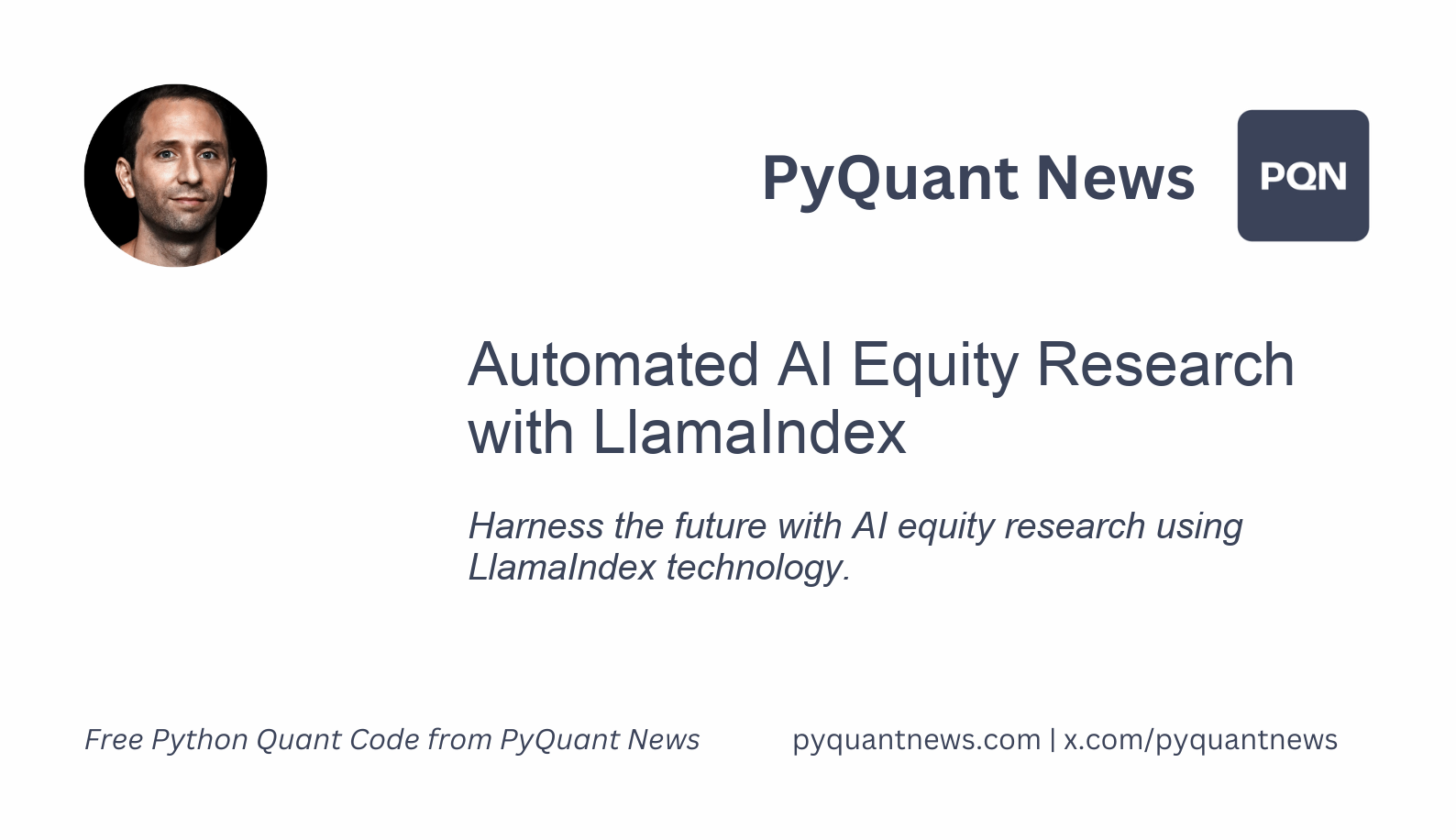
Automated AI Equity Research with LlamaIndex
Introduction
In financial markets today, success relies not just on information, but on the swift processing of immense data volumes. Traditional equity research methods are evolving as artificial intelligence and automated AI financial agents play a transformative role. At the heart of this transformation is LlamaIndex technology, which offers significant advancements in financial data processing. This article delves into how LlamaIndex can be utilized to develop automated AI equity research agents, and examines its impact on the finance sector.
Understanding LlamaIndex
LlamaIndex is a potent tool for indexing and retrieving data, offering a streamlined way to organize complex information. In the ever-expanding world of financial datasets, LlamaIndex technology provides unmatched precision and speed. For AI integration in finance, particularly in equity research, its capability to handle extensive data volumes significantly enhances decision-making processes. LlamaIndex thus stands as a cornerstone for developing any advanced equity research agent.
Redefining Equity Research with Automation
The Traditional Approach
Historically, equity research demanded intensive manual labor, with experts examining market trends and financial statements for hours on end. As the magnitude of data increases, the inefficiency of manual processes becomes evident. The shift towards AI integration in finance is not just beneficial—it is necessary, offering unprecedented speed and accuracy.
The AI Advantage
AI-powered systems excel in analyzing large datasets swiftly, uncovering insights that might escape the human eye. Tools such as natural language processing in finance and deep learning for stock prediction provide detailed analytical capabilities. In equity research, they transform complex data into actionable insights in real time.
Unique Role of LlamaIndex
LlamaIndex technology revolutionizes data acquisition and processing, offering seamless access to both historical and real-time financial data necessary for AI models. Its robust APIs ensure smooth data handling, which is critical for feeding accurate information into AI-driven investment strategies.
Building the AI Equity Research Agent
Step 1: Data Acquisition and Preprocessing
High-quality data is foundational to effective AI models. LlamaIndex excels in acquiring and structuring this data, making it ideal for AI applications. The technology's APIs provide an efficient pathway for normalizing and cleaning data, which optimizes it for machine learning.
Step 2: Integrating AI Models
The core of an AI equity research agent lies in the models it uses. By applying natural language processing and deep learning, these agents can interpret complex financial reports and predict market trends. LlamaIndex enhances these processes, ensuring the efficient indexing of complex financial data, thereby boosting both accuracy and analytical depth.
Step 3: Implementing Sentiment Analysis
Market sentiment analysis is integral to comprehensive equity research. Sentiment analysis tools evaluate news, social media, and reports, offering insights into investor psychology and potential market movements. LlamaIndex supports this by indexing sentiment-rich data, which is fundamental for informed investment decisions.
Step 4: Conducting Backtesting and Validation
Prior to deployment, rigorous backtesting is vital to determine predictive accuracy. LlamaIndex assists in this by indexing diverse historical data, ensuring robust testing. High accuracy levels reduce investment risks, making predictions more reliable.
Step 5: Deployment and Monitoring
Once developed, deploying the AI equity research agent into the financial ecosystem is streamlined by LlamaIndex's support for scalable deployment. Continuous monitoring is key to keeping up with market dynamics, with feedback loops refining models post-deployment to maintain their cutting-edge performance.
Ethical Considerations in AI Finance
As AI systems become more prevalent in finance, ethical considerations rise to the forefront. Ensuring transparent algorithms and regular audits can mitigate biases in decision-making. LlamaIndex, along with similar technologies, must embed ethical safeguards aligning with sustainable financial practices, promoting responsible AI in finance.
The Future of Equity Research
The integration of AI in equity research signals a promising future where AI agents not only predict trends but also tailor investment strategies to individual preferences. As the focus on ethical AI intensifies, these agents could drive the adoption of green and sustainable investments. LlamaIndex is ideally placed to adapt to these progressive AI applications, supporting sustainable and informed financial growth.
Resources for Further Exploration
For those seeking to deepen their understanding of this field, the following resources are invaluable: LlamaIndex's official documentation, Yves Hilpisch's book "Artificial Intelligence in Finance," Coursera’s specialization in "Machine Learning for Finance," Kaggle's financial data competitions, and the "AI for Finance Podcast."
Conclusion
AI integration in finance is opening a new era for equity research. Leveraging LlamaIndex technology enriches data precision and improves access to insights, ushering in innovative methods for investment strategies. By embracing these changes, the financial market can remain informed, accessible, and sustainable in the digital age.